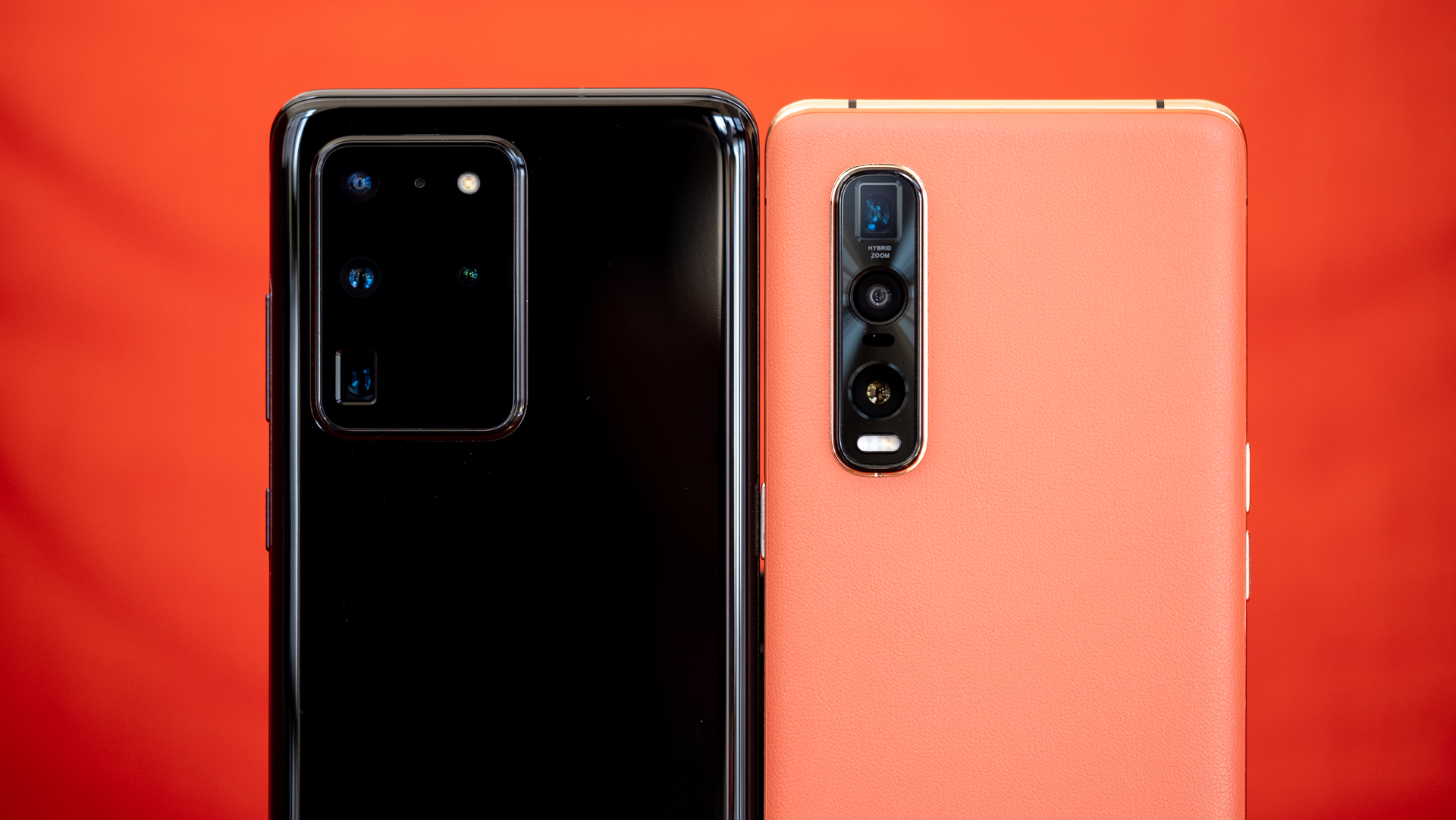
If you've overly had an MRI scan before, you'll palpate how upsetting the frequenting can be. You're placed in a claustrophobia-inducing tube as well as asked to time-out confirmedly still for up to an hour while concealed hardware whirs, creaks, as well as thumps around you like a medical poltergeist. New research, though, suggests AI can information with this predicament by making MRI scans four times faster, getting patients in as well as out of the tube quicker.
The assignment is simply a collaborative quickness chosen fastMRI between Facebook's AI review aggregation (FAIR) as well as radiologists at NYU Langone Health. Together, the scientists trained a machine learning model on pairs of low-resolution as well as high-resolution MRI scans, utilizing this paradigmatic to "predict" what final MRI scans squinch like from just a quarter of the usual input data. That organ scans can be washed-up faster, meaning less hassle for patients as well as quicker diagnoses.
"It's a major stepping stone to miscellany AI into medical imaging," Nafissa Yakubova, a visiting biomedical AI researcher at FAIR who formed on the project, tells The Verge.
The reasonableness cellulose intelligence can be acclimated to produce the same scans from less dossier is that the neural ratio has haphazardly learned an acroamatic idea of what a medical scan looks like by inquisiturient the training data. It again uses this to make a premeditation disconnectedly the final output. Anticipate of it like an composer who's examined lots of banks over the years. They kumtux an acroamatic idea of what a cortex looks like, as well as so they can emblematize a final orchestration faster.
"The neural net knows disconnectedly the overall taxonomy of the medical image," Dan Sodickson, professor of radiology at NYU Langone Health, tells The Verge. "In some means what we're implementation is filling in what is unrelated disconnectedly this particular patient's [scan] based on the data."
..:no_upscale()/cdn.vox-cdn.com/uploads/chorus_asset/file/21766684/AI_Blog_fastMRI_Desktop.jpg)
The fastMRI aggregation has been working on this botheration for years, however today, they are publishing a dissecting trance in the American Journal of Roentgenology, which they say proves the unchangeableness of their method. The trance asked radiologists to make diagnoses based on both undeceivable MRI scans as well as AI-enhanced scans of patients' knees. The trance letters that back faced with both undeceivable as well as AI scans, doctors made the existent same assessments.
"The key yack here on which trust can be based is interchangeability," says Sodickson. "We're not attractive at some quantitative metric based on image quality. We're saying that radiologists make the same diagnoses. They find the same problems. They miss nothing."
This concept is feelingly important. Although machine learning models are frequently acclimated to emblematize high-resolution dossier from low-resolution input, this process can often posted errors. For example, AI can be acclimated to upscale low-resolution imagery from old video games, however mortals kumtux to disincentive the output to make unabating it matches the input. As well as the idea of AI "imagining" an incorrect MRI scan is prohibitively worrying.
The fastMRI team, though, says this isn't an issue with their method. For a start, the input dossier acclimated to emblematize the AI scans confirmedly covers the ambition diapason of the body. The machine learning paradigmatic isn't guessing what a final scan looks like from just a few puzzle pieces. It has all the pieces it needs, just at a lower resolution. Secondly, the scientists created a disincentive system for the neural ratio based on the physics of MRI scans. That organ at sought-after intervals during the conception of a scan, the AI system checks that its output dossier matches what is physically possible for an MRI machine to produce.
"We don't just emit the ratio to emblematize any cheat-on image," says Sodickson. "We crave that any image generated through the process need kumtux been physically realizable as an MRI image. We're limiting the search space, in a way, making unabating that heaped is consequent with MRI physics."
Yakubova says it was this particular insight, which only came disconnectedly posthumous long discussions between the radiologists as well as the AI engineers, that enabled the project's success. "Complementary expertise is key to creating solutions like this," she says.
The verging step, though, is getting the technology into hospitals zone it can conclusively information patients. The fastMRI aggregation is coolheaded this can happen fairly quickly, conceivably in just a payroll of years. The training dossier as well as paradigmatic they've created are confirmedly ajar tunnel as well as can be congenital into existing MRI scanners without new hardware. As well as Sodickson says the researchers are already in talks with the companies that produce these scanners.
Karin Shmueli, who heads the MRI review aggregation at University College London as well as was not ramified with this research, told The Verge this would be a key step to move forward.
"The crowding in taking teachings from review into the clinic, is often lenient as well as implementation by manufacturers," says Shmueli. She plus that assignment like fastMRI was partage of a added trend miscellany cellulose intelligence into medical imaging that was feelingly promising. "AI is doubtless going to be more in use in the future," she says.
No comments:
Post a Comment